Minimum-Cost Reach Avoid Problem
We tackle the problem of solving minimum-cost reach avoid problems. That is, we wish to minimize the cumulative cost to reach the goal while avoiding collision.
Unlike normal MDPs, the minimum-cost reach avoid problem has the following differences:
- The time horizon \(T\) is a decision variable
- Unlike the safety constraint, the goal constraint is only enforced at the terminal timestep T
These differences prevent the straightforward application of existing RL methods to solve this problem.
Experiments
We compare RC-PPO on six minimum-cost reach-avoid environments.
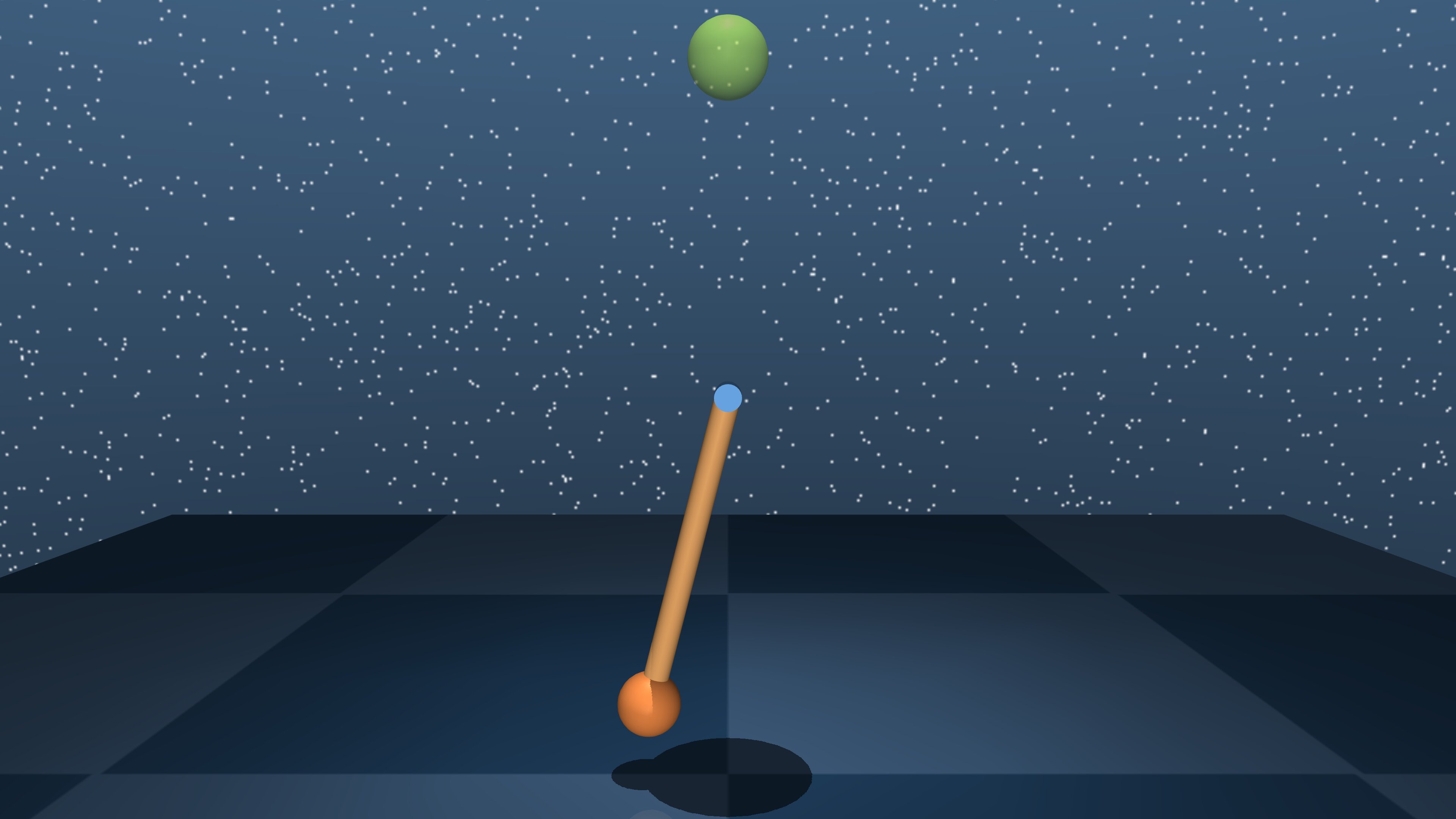
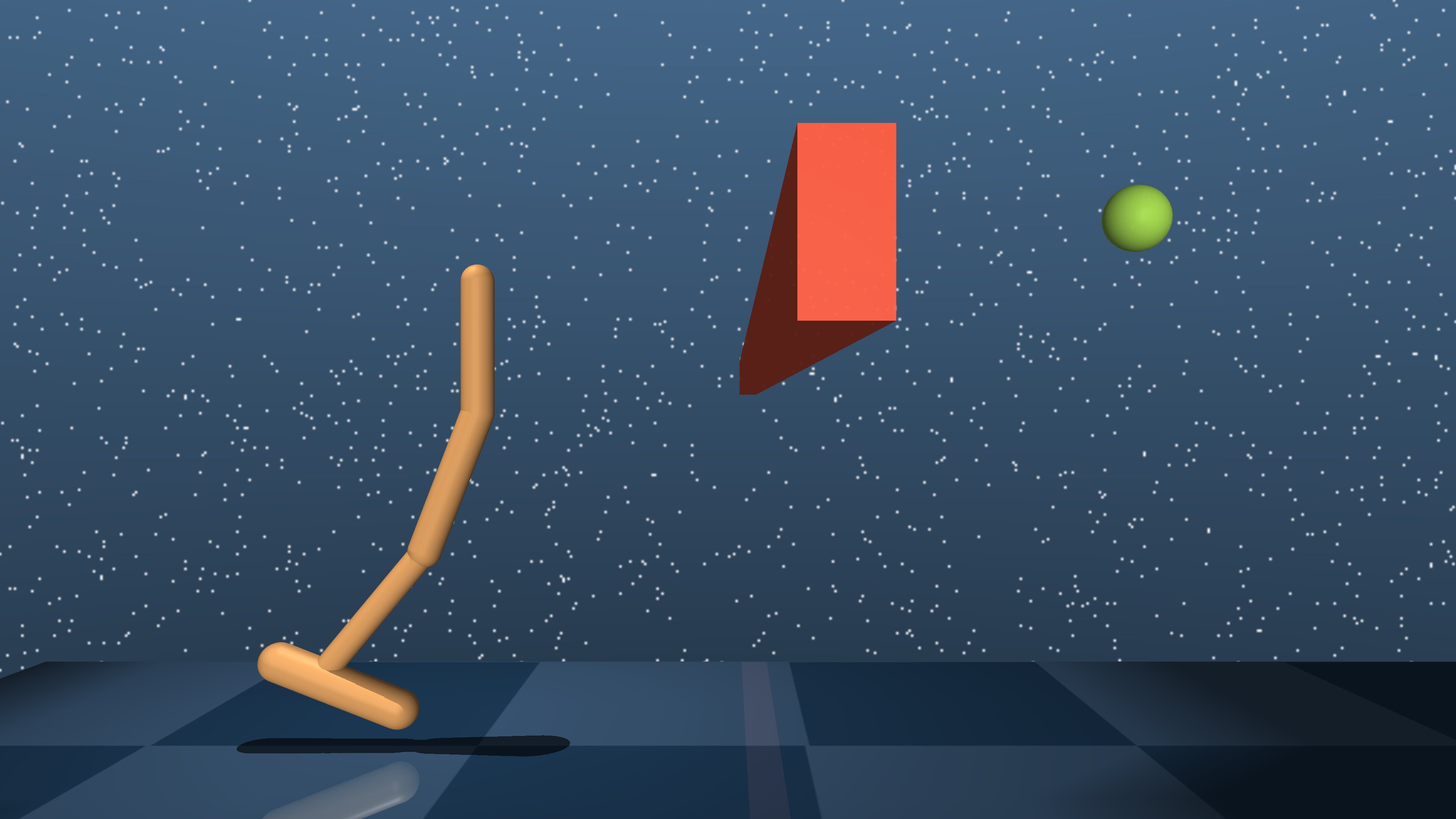
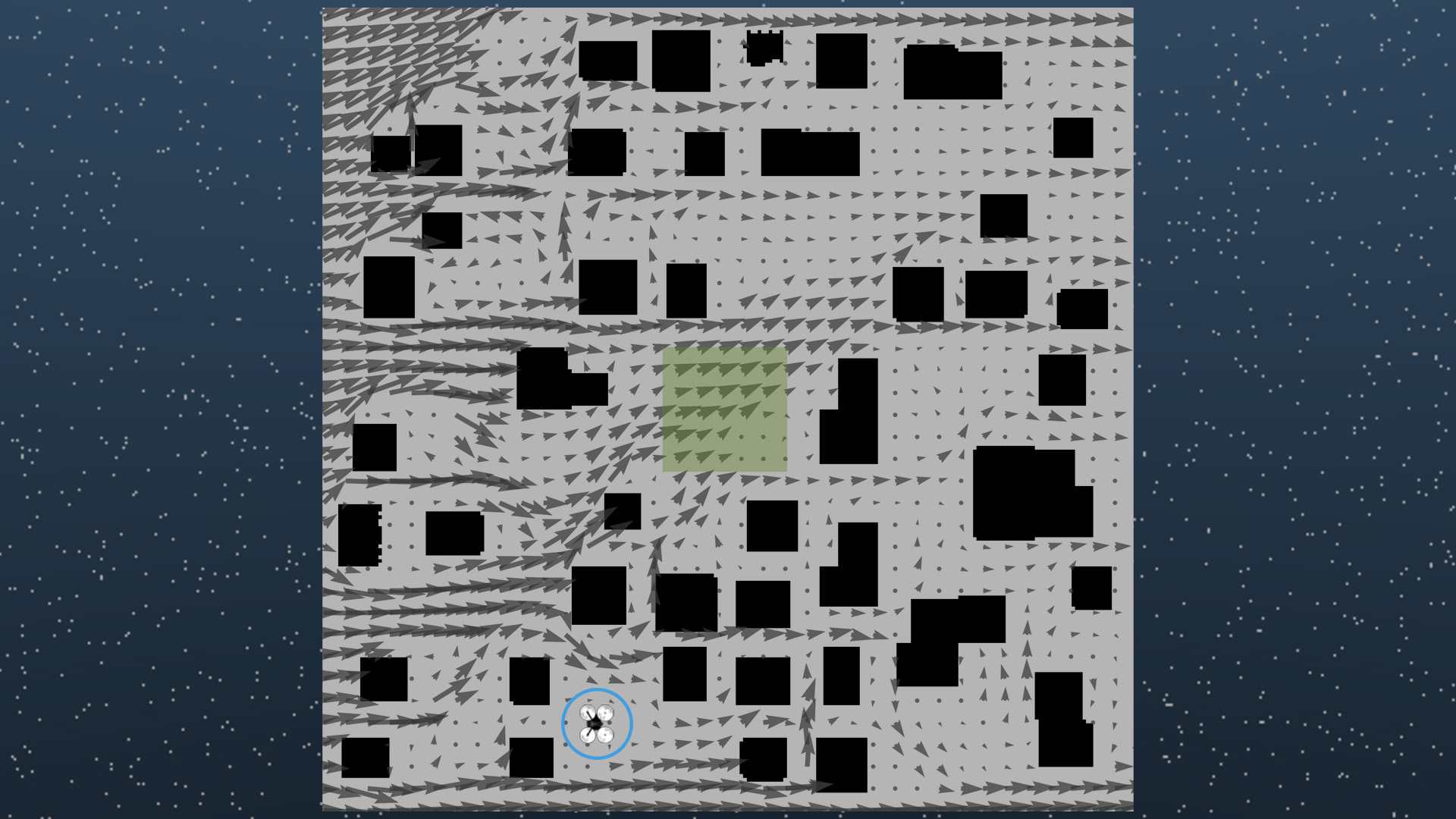
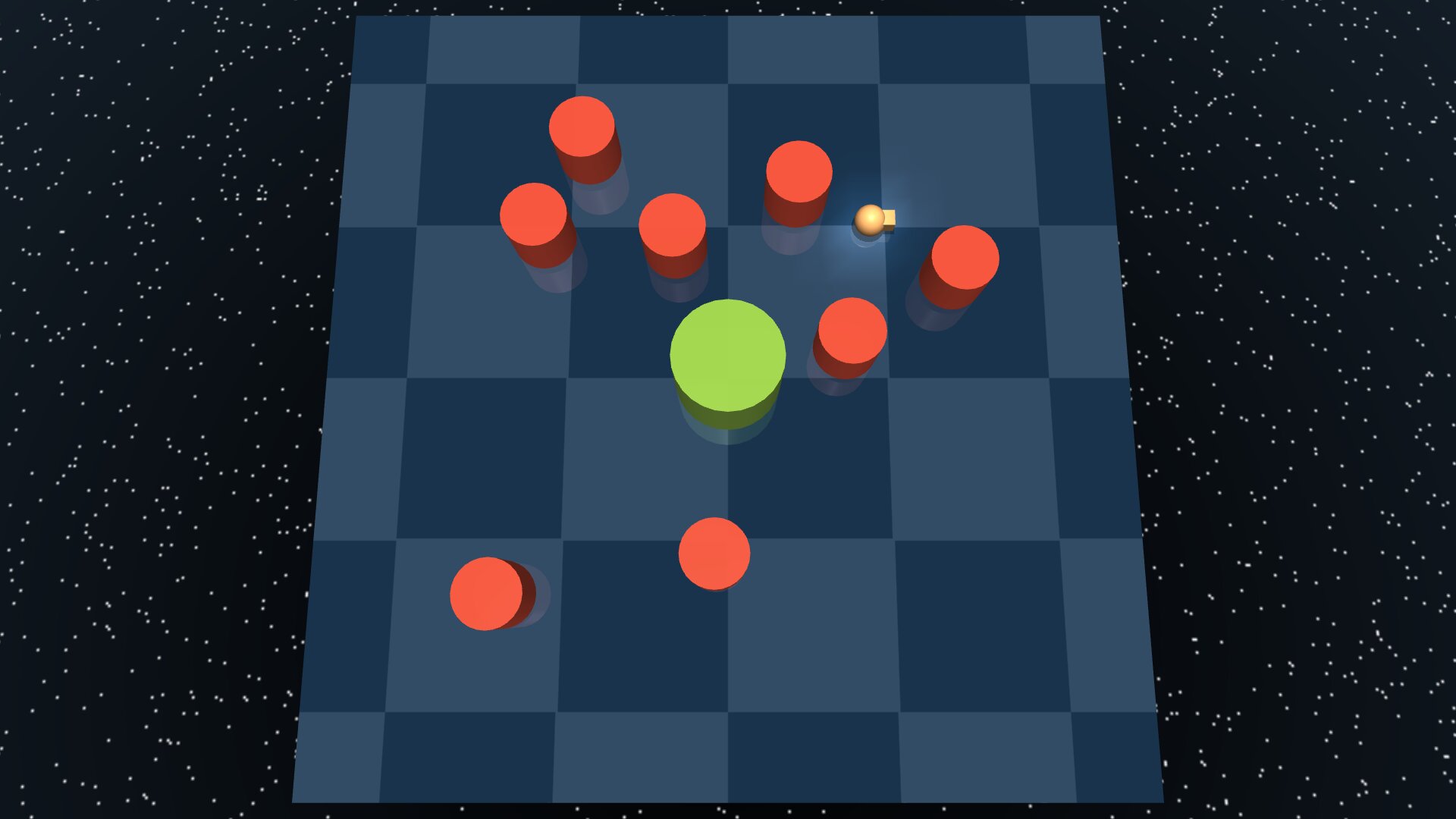
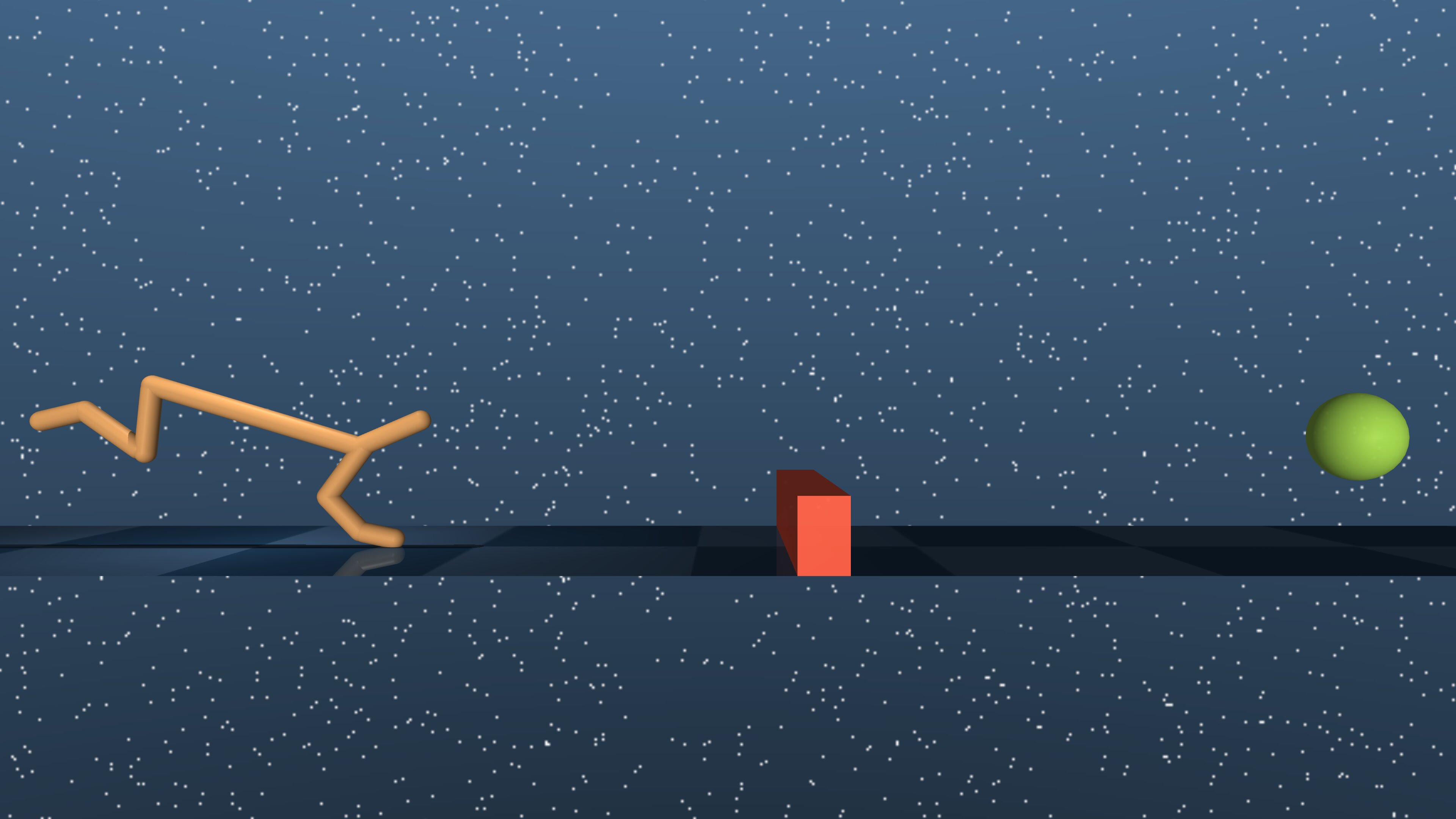
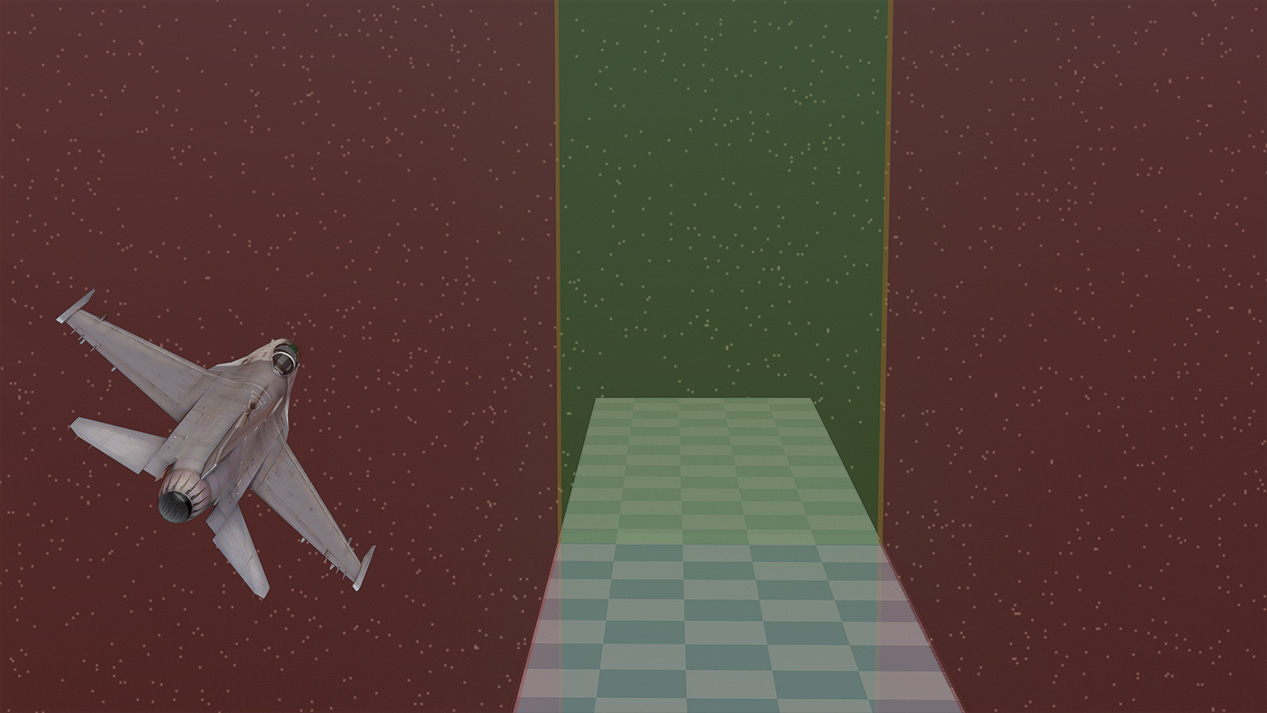
For each task, we compare the interquartile mean (IQM) of the cumulative and the reach rates of the final converged policies. RC-PPO achieves significantly lower costs while retaining comparable reach rates even when compared to the best baselines that use reward-shaping.
Abstract
Current reinforcement-learning methods are unable to directly learn policies that solve the minimum cost reach-avoid problem to minimize cumulative costs subject to the constraints of reaching the goal and avoiding unsafe states, as the structure of this new optimization problem is incompatible with current methods. Instead, a surrogate problem is solved where all objectives are combined with a weighted sum. However, this surrogate objective results in suboptimal policies that do not directly minimize the cumulative cost.
In this work, we propose RCPPO, a reinforcement-learning-based method for solving the minimum-cost reach-avoid problem by using connections to Hamilton-Jacobi reachability. Empirical results demonstrate that RCPPO learns policies with comparable goal-reaching rates to while achieving up to 57% lower cumulative costs compared to existing methods on a suite of minimum-cost reach-avoid benchmarks on the Mujoco simulator.